Data science has a profound impact on social media by enabling sophisticated audience segmentation and targeted advertising strategies. Utilizing machine learning algorithms, social media platforms can analyze user behaviors and preferences to tailor content that resonates on an individual level. Sentiment analysis provides real-time insights into public opinion, allowing brands to adapt their strategies proactively.
This integration of data science not only enhances user engagement but also maximizes advertising effectiveness, ensuring a higher return on investment. However, the implications of these advancements extend far beyond mere marketing tactics, prompting an exploration into the broader consequences for privacy and user autonomy.
Key Takeaways
- Data science categorizes users for personalized marketing strategies based on demographics, interests, and behaviors.
- Machine learning algorithms analyze user data to create targeted advertising campaigns that enhance ad relevance and conversion rates.
- Sentiment analysis tracks real-time public opinion, allowing for responsive decision-making and reputation management.
- Performance metrics, such as engagement and conversion rates, are analyzed to optimize influencer marketing strategies.
- Competitive analysis and real-time alerts help refine tactics and identify growth opportunities in social media marketing.
User Segmentation in Social Media
User segmentation in social media leverages data science to categorize users into distinct groups based on demographics, interests, and behaviors, thereby optimizing targeted marketing strategies. This methodical approach allows businesses to dissect vast pools of user data and identify segments with shared characteristics and preferences. By doing so, companies can fine-tune their marketing messages to align more closely with the specific needs and interests of each segment.
Data-driven user segmentation enhances the personalization of targeted marketing campaigns, increasing engagement and conversion rates. Through advanced analytical techniques, data science can sift through metrics such as age, location, gender, online activity, and past purchasing behavior. This data-centric methodology enables marketers to understand their audience at a granular level, ensuring content resonates more profoundly with each user group.
Precise user segmentation facilitates the creation of tailored content and messaging. Instead of adopting a one-size-fits-all approach, businesses can deliver unique experiences to different audience segments. This targeted approach not only drives higher engagement but also guarantees that marketing efforts are directed towards the most relevant and receptive audience groups.
Ultimately, data-driven user segmentation in social media is instrumental in maximizing the effectiveness and efficiency of marketing strategies.
Targeted Advertising in Social Media
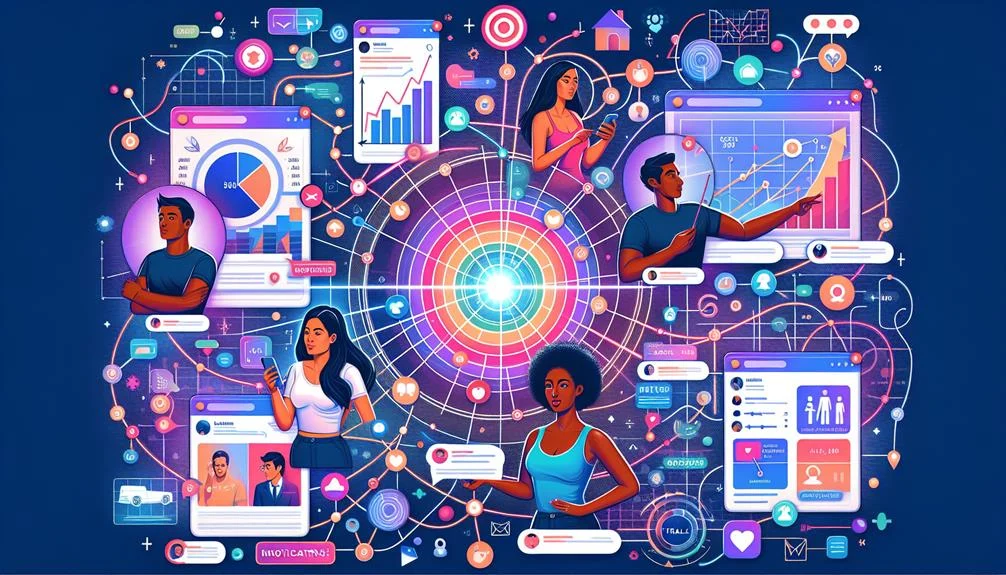
Targeted advertising leverages data science to analyze user behavior, allowing for precision in reaching specific audience segments. By employing sophisticated ad personalization techniques, businesses can tailor advertisements to align with individual user preferences and actions.
This methodical approach not only enhances ad relevance but also optimizes marketing budgets and improves conversion rates.
User Behavior Analysis
Frequently, data science leverages the intricate analysis of social media activity to optimize targeted advertising campaigns by fully understanding consumer preferences, interests, and behaviors. By meticulously analyzing social media interactions, data science facilitates a thorough user behavior analysis, which is pivotal in crafting more effective advertising strategies.
This analytical approach helps in predicting potential customer responses and tailoring messages that resonate with specific audience segments, thereby enhancing the relevance and impact of advertising campaigns.
To illustrate, consider the following table highlighting key aspects of user behavior analysis in targeted advertising:
Aspect | Description | Impact on Advertising Campaigns |
---|---|---|
Data Collection | Gathering social media engagement data | Forms the foundation for accurate analysis |
Behavioral Patterns | Identifying trends and preferences | Helps in creating tailored ad content |
Predictive Analytics | Forecasting user responses | Increases ad relevance and engagement |
ROI Optimization | Adjusting strategies based on insights | Improves return on investment (ROI) |
Ad Personalization Techniques
Building upon the insights gained from user behavior analysis, ad personalization techniques leverage data science to create highly targeted advertising strategies that resonate with individual preferences and interests.
Utilizing machine learning algorithms, these techniques analyze vast datasets encompassing user behavior, demographics, and interests on social media platforms. The primary objective is to match relevant ads with users’ specific characteristics, thereby increasing engagement and conversion rates.
Machine learning plays a pivotal role in predicting user preferences, allowing for the delivery of tailored ads. By continuously analyzing user interactions, these algorithms adapt and refine their predictions, ensuring that the ads presented are both timely and relevant. This constant optimization is vital for maintaining high levels of ad performance on social media.
Moreover, targeted advertising driven by data analysis ensures ad relevance, leading to a significant increase in return on investment (ROI) for businesses. By focusing on users most likely to engage with the content, companies can allocate their advertising budgets more efficiently, reducing wastage and maximizing impact.
Essentially, ad personalization through data science not only enhances user experience but also drives business success by creating a symbiotic relationship between advertisers and consumers.
Sentiment Analysis
Sentiment analysis in social media employs emotion detection techniques to categorize user-generated content, enabling real-time sentiment tracking across various platforms. By leveraging these techniques, businesses can gain immediate insights into public opinion and adjust their strategies accordingly.
This capability profoundly impacts brand strategy, allowing for more responsive and informed decision-making.
Emotion Detection Techniques
Emotion detection techniques, particularly sentiment analysis, leverage natural language processing (NLP) to systematically classify social media content as positive, negative, or neutral, thereby enabling the precise measurement of user sentiments. These techniques are vital in data science, where extracting actionable insights from social media data is essential for understanding public opinion and brand perception.
Data science employs advanced algorithms to dissect user-generated content, identifying key emotions such as joy, anger, sadness, and surprise. Sentiment analysis operates by parsing the text for emotional cues, evaluating context, and applying machine learning models to categorize the sentiment accurately. This methodical approach ensures that businesses can decipher nuanced customer feedback and adapt their strategies accordingly.
Emotion detection tools are invaluable for businesses aiming to refine their products and tailor their marketing strategies. By understanding the emotional undertones in social media discussions, companies can promptly address customer concerns, enhance user experience, and foster brand loyalty.
Additionally, sentiment analysis is instrumental in crisis management, as it allows organizations to monitor and respond to shifts in public sentiment during events or controversies, thereby mitigating potential reputational damage. Therefore, emotion detection techniques represent a cornerstone of effective social media analytics.
Real-Time Sentiment Tracking
Leveraging advanced algorithms, real-time sentiment tracking in social media enables businesses to swiftly detect and respond to shifts in public opinion. This is achieved through sentiment analysis, a data science technique that processes text data to determine whether the sentiment expressed in social media posts is positive, negative, or neutral. By employing Natural Language Processing (NLP), these sophisticated algorithms can analyze large volumes of social media content with high accuracy and speed.
Real-time tracking of sentiment allows organizations to continuously monitor brand perception and public opinion on various topics. This continuous analysis helps in identifying emerging trends in customer sentiment, which can be pivotal for timely decision-making. For instance, a sudden spike in negative sentiment can alert businesses to potential issues that may require immediate attention, thereby mitigating risks before they escalate.
Categorizing sentiments in real-time, companies can better understand the effectiveness of their communication strategies and customer engagement efforts. This data-driven approach enables businesses to make informed adjustments to their marketing strategies, ensuring they remain aligned with public sentiment and enhance customer satisfaction.
Impact on Brand Strategy
Understanding consumer sentiment through data science is essential for refining brand strategies and enhancing overall customer satisfaction. By leveraging sentiment analysis, brands can systematically evaluate vast amounts of social media data to classify opinions as positive, negative, or neutral. This rigorous approach offers actionable insights, enabling companies to tailor their marketing strategies and respond adeptly to consumer feedback.
Sentiment analysis tools, powered by advanced analytics, process and interpret large volumes of social media data, providing a nuanced understanding of public perception. Utilizing these tools, brands can:
- Identify Consumer Trends: Track sentiment trends over time to discern shifts in consumer attitudes, helping to anticipate market changes and stay ahead of competitors.
- Enhance Customer Engagement: Adjust marketing strategies based on real-time social media feedback, fostering stronger relationships with customers and enhancing brand loyalty.
- Improve Product Offerings: Analyze sentiment data to pinpoint areas for product improvement, aligning offerings with consumer needs and preferences.
- Reputation Management: Monitor public sentiment to manage brand reputation proactively, addressing negative feedback swiftly to mitigate potential damage.
Influencer Marketing
Influencer marketing, powered by data science, enables brands to meticulously identify and collaborate with key influencers to effectively reach and engage their target audience. Data science tools are pivotal in analyzing performance metrics such as engagement rates and conversions, thereby providing data-driven insights that optimize influencer partnerships. These insights enable brands to select influencers whose audience demographics align closely with their target market, ensuring maximum relevancy and impact.
Utilizing data science, brands can track and measure various performance metrics to evaluate the effectiveness of influencer campaigns. This methodical approach allows for a detailed analysis of engagement patterns, helping brands to make informed decisions and refine their strategies for best results. Consequently, the strategic use of data science in influencer marketing not only enhances brand visibility but also improves audience engagement and ROI.
Metric | Description | Benefit |
---|---|---|
Engagement Rates | Measures likes, comments, shares | Indicates influencer-audience interaction |
Conversion Rates | Tracks actions taken post-engagement | Evaluates campaign effectiveness |
ROI | Calculates financial return from the campaign | Assesses overall profitability |
Ultimately, data science empowers brands to forge successful influencer collaborations, ensuring that marketing efforts are both efficient and impactful. By continuously analyzing data from influencer campaigns, brands can adapt and evolve their strategies, maintaining a competitive edge in the dynamic social media landscape.
Performance Monitoring
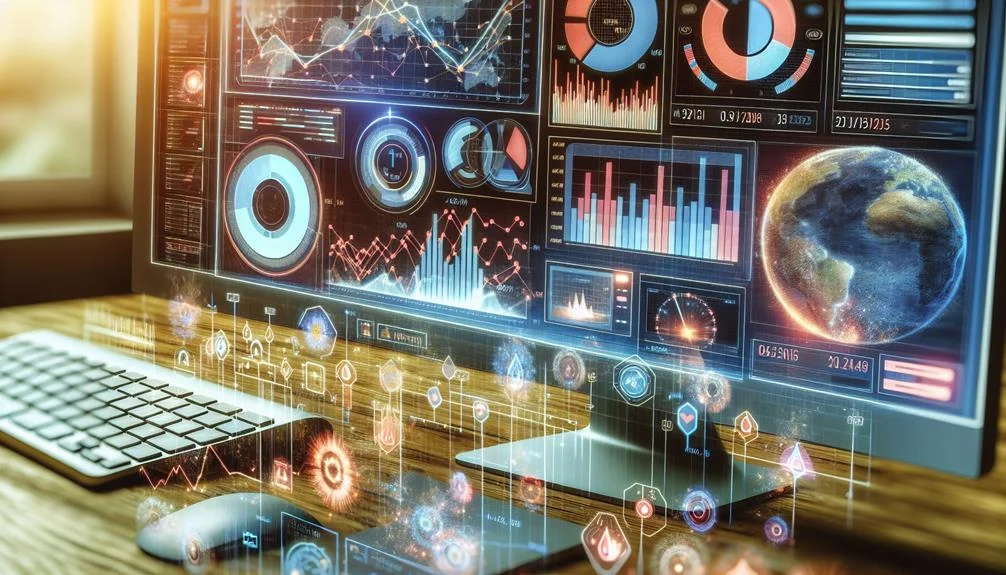
Building upon the strategic insights gained from influencer marketing, performance monitoring in social media leverages data science to provide real-time analytics on key metrics such as click-through rates and conversions. This analytical approach allows platforms and advertisers to continuously track and adjust their campaigns, ensuring best outcomes and efficient resource utilization.
Key aspects of performance monitoring include:
- Click-Through Rates (CTR):
By tracking CTR, data science helps determine the effectiveness of ads in engaging users. Real-time monitoring of CTR allows for immediate adjustments to improve ad performance.
- Conversions:
Monitoring conversion rates provides insights into how many users complete a desired action, such as making a purchase or signing up for a newsletter. This metric is essential for evaluating the return on investment (ROI) of social media campaigns.
- Impressions:
Keeping track of how many times an ad is displayed helps in understanding its reach. By analyzing impression data, advertisers can gauge the visibility of their campaigns and make data-informed decisions.
- Resource Allocation:
Real-time monitoring of these key metrics enables more effective allocation of resources. Advertisers can identify underperforming ads and reallocate budgets to those yielding better results.
Competitive Analysis in Social Media
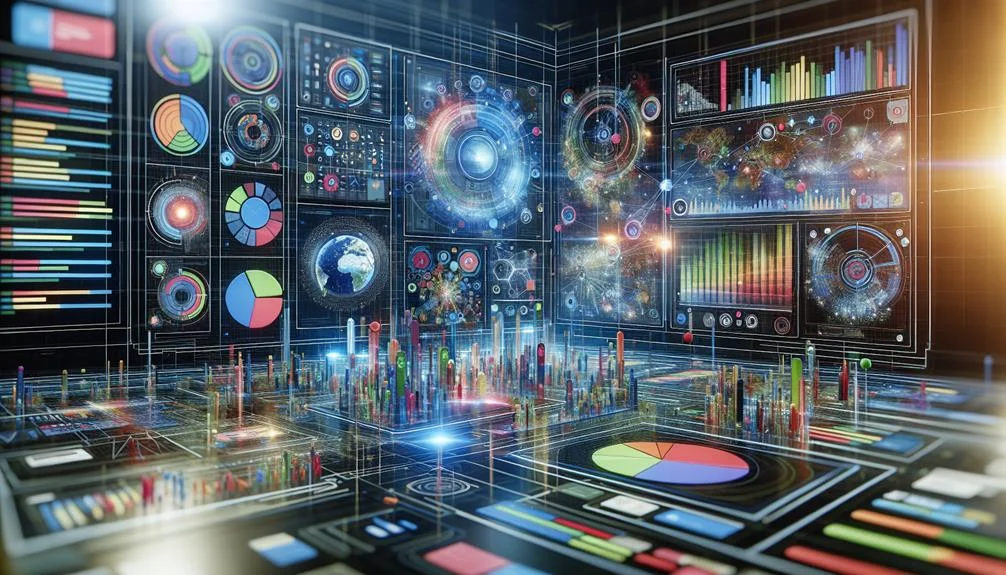
Competitive analysis in social media leverages data science to meticulously track and evaluate competitors’ content performance, audience engagement, and advertising strategies. By harnessing data analytics, businesses can obtain a granular understanding of how competitors’ social media efforts resonate with their audience. This involves monitoring metrics such as likes, shares, comments, and overall engagement rates, as well as the effectiveness of various advertising campaigns.
Data science tools facilitate the extraction of insights regarding competitors’ audience demographics, preferences, and sentiments. These insights enable businesses to identify emerging trends, market gaps, and new opportunities for growth. For instance, sentiment analysis can reveal the emotional responses of audiences to competitors’ content, providing a benchmark for refining one’s own social media strategies.
Through competitive analysis, organizations can benchmark their performance against industry standards and refine their tactics to enhance content quality, engagement, and advertising efficiency. The iterative nature of data analytics ensures that businesses can stay agile and responsive to market dynamics.
Real-time Alerts
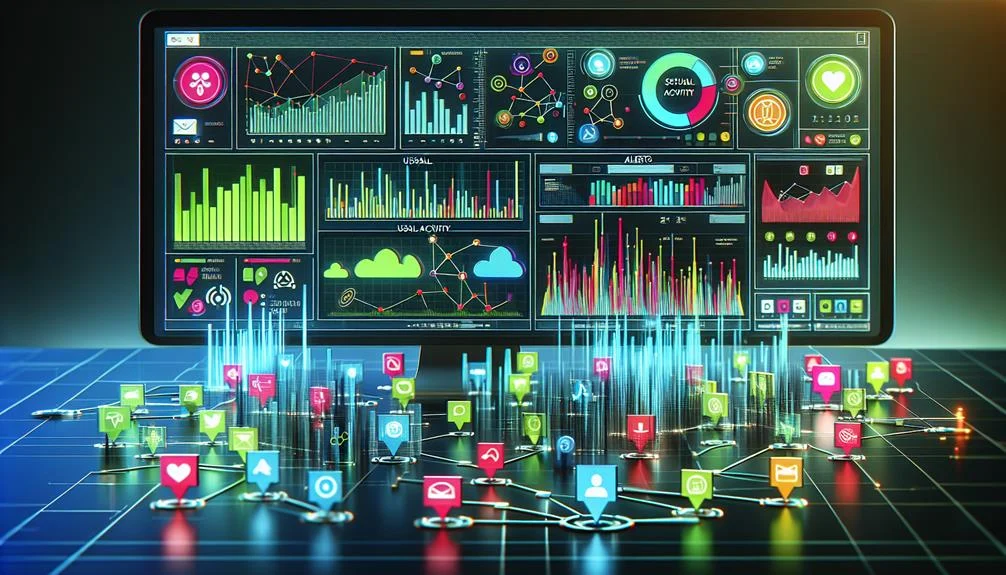
In addition to benchmarking performance through competitive analysis, real-time alerts powered by data science enable businesses to monitor brand mentions and customer interactions instantaneously. By leveraging sophisticated algorithms, companies can analyze social media data to detect trends, sentiments, and potential crises as they emerge. This proactive approach allows organizations to respond swiftly to customer feedback, engage meaningfully with followers, and manage their online reputation more effectively.
Real-time alerts utilize data science to provide timely notifications, which are essential for proactive social media management. These alerts can be tailored to notify businesses about specific keywords, hashtags, or competitor activities.
Here are four key ways real-time alerts benefit businesses:
- Swift Response to Customer Feedback: Immediate alerts about customer comments or complaints allow companies to address issues promptly, improving customer satisfaction and loyalty.
- Crisis Management: Early detection of negative sentiments or potential crises enables businesses to mitigate damage by responding quickly and appropriately.
- Engagement Opportunities: Real-time notifications about trending topics or positive mentions help businesses capitalize on opportunities to engage with their audience.
- Competitive Monitoring: Alerts about competitor activities or industry trends keep businesses informed, enabling them to adjust their strategies accordingly.
Conclusion
A striking statistic reveals that targeted advertising driven by data science can boost ad engagement by up to 50%. This underscores the transformative potential of data science in social media, where user segmentation, sentiment analysis, and real-time alerts collectively enhance marketing strategies.
By leveraging machine learning algorithms and advanced analytics, businesses achieve higher ROI and more effective audience engagement, demonstrating the integral role of data science in modern digital marketing.