Integrating data science in healthcare has transformed the landscape of patient care and medical research. By leveraging the power of data-driven algorithms, healthcare professionals can now explore areas of disease detection, treatment optimization, and personalized patient interventions with unprecedented precision.
Using machine learning models and predictive analytics has enhanced diagnostic capabilities and paved the way for groundbreaking advancements in pharmaceutical research and population health management. The impact of data science in healthcare is profound and multifaceted, touching every aspect of the industry with its potential for innovation and improvement.
Key Takeaways
- Automates image analysis for disease detection and early intervention.
- Optimizes drug development, dosage, and adverse reaction identification.
- Predicts health outcomes and tailors interventions for at-risk populations.
- Tracks diseases, forecasts outbreaks, and guides public health policies.
- Manages patient health records for seamless care coordination and improved services.
Data Science in Healthcare: Medical Imaging Applications
Data science plays a pivotal role in healthcare through its medical imaging applications, revolutionising how diseases and conditions are diagnosed and treated.
In medical imaging applications, data science-driven algorithms are instrumental in analyzing images to detect abnormalities, tumors, fractures, and other health issues with remarkable accuracy. Machine learning models further enhance the capabilities of radiologists by aiding in the interpretation of images swiftly and with heightened precision. This not only expedites the diagnostic process but also leads to improved patient outcomes.
The integration of data science in medical imaging allows for automation of image analysis, reducing the likelihood of human error and expediting the identification of potential health concerns. Particularly significant is the role of data science in facilitating early detection of diseases like cancer.
This early detection paves the way for timely treatment interventions, ultimately improving prognosis and quality of life for patients.
Data Science in Healthcare: Pharmaceutical Development Insights
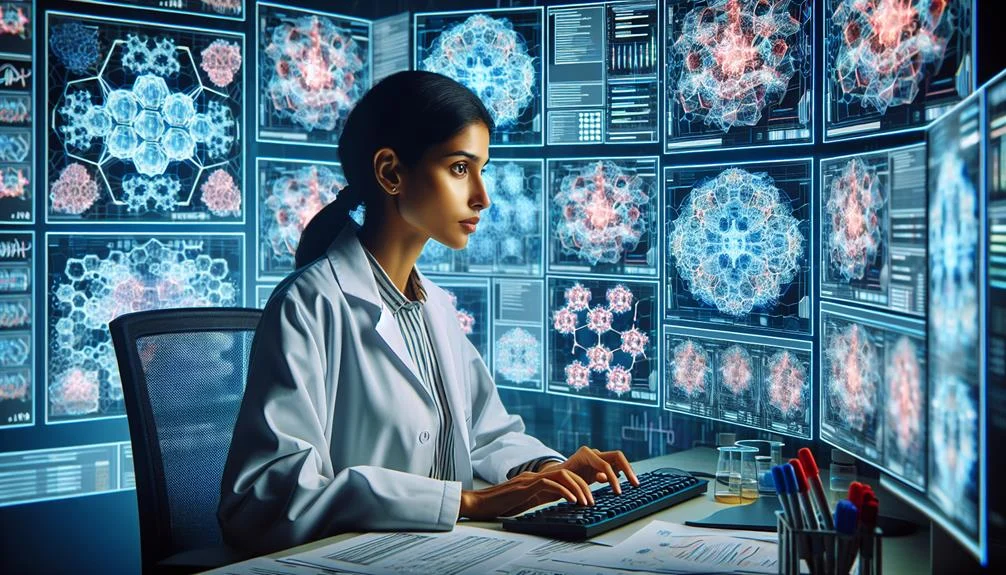
Pharmaceutical development insights provide critical analysis and strategic guidance for optimizing medication effectiveness, predicting drug interactions, and enhancing drug safety profiles in the healthcare industry.
Data science plays a pivotal role in pharmaceutical research. It delves into molecular structures, biological pathways, and drug mechanisms to discover potential treatments. By analyzing vast datasets, it aids in identifying adverse reactions, optimizing dosages, and tailoring drug regimens for personalized medicine based on individual genetic profiles.
Data science supports the acceleration of clinical trials, enabling pharmaceutical companies to bring innovative medications to market more efficiently. By leveraging data-driven insights, researchers can enhance drug safety profiles, mitigate risks of drug interactions, and improve overall patient outcomes.
The integration of data science in pharmaceutical development streamlines drug discovery processes and contributes to the advancement of effective and safe medications in healthcare.
Predictive Modeling in Healthcare
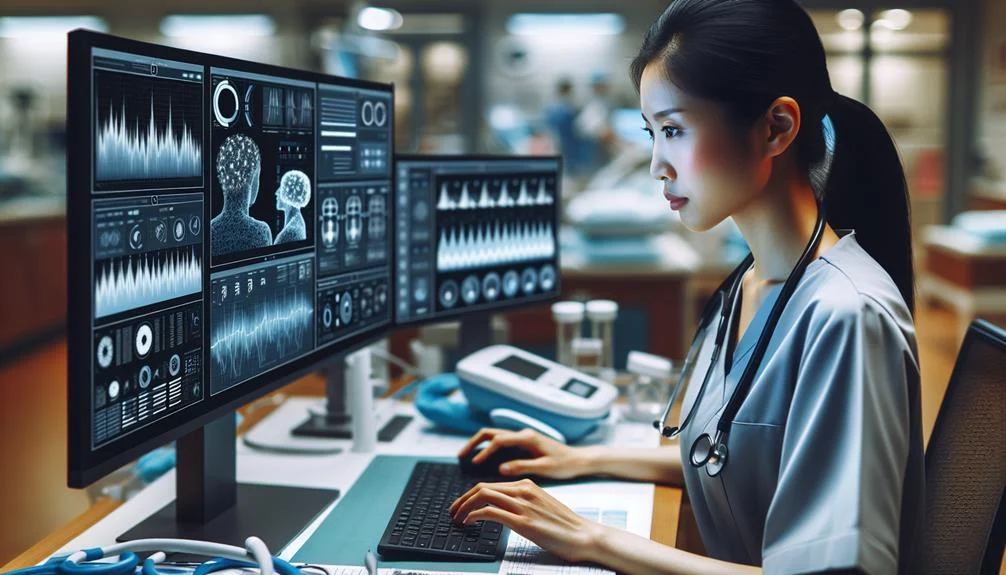
How can predictive modeling revolutionize healthcare by leveraging data science to forecast future health outcomes and optimize patient care strategies? Predictive modeling in healthcare utilizes machine learning algorithms to analyze historical data and predict potential health outcomes. By identifying at-risk populations and enabling proactive interventions, healthcare providers can deliver personalized patient care and improve healthcare quality. Through the utilization of predictive modeling, healthcare organizations can forecast patient readmissions, medication adherence, and disease progression, ultimately leading to more informed decision-making processes.
Benefits of Predictive Modeling in Healthcare |
---|
Identifying at-risk populations |
Enabling proactive interventions |
Improving healthcare quality |
Predictive modeling optimizes resource allocation, enhances treatment strategies, and prioritizes preventive care initiatives, ultimately leading to better patient outcomes and reduced healthcare costs. By embracing predictive modeling, healthcare providers can improve their ability to forecast future health outcomes and tailor their interventions to meet individual patient needs more effectively.
Data Science in Healthcare: Disease Tracking and Prevention
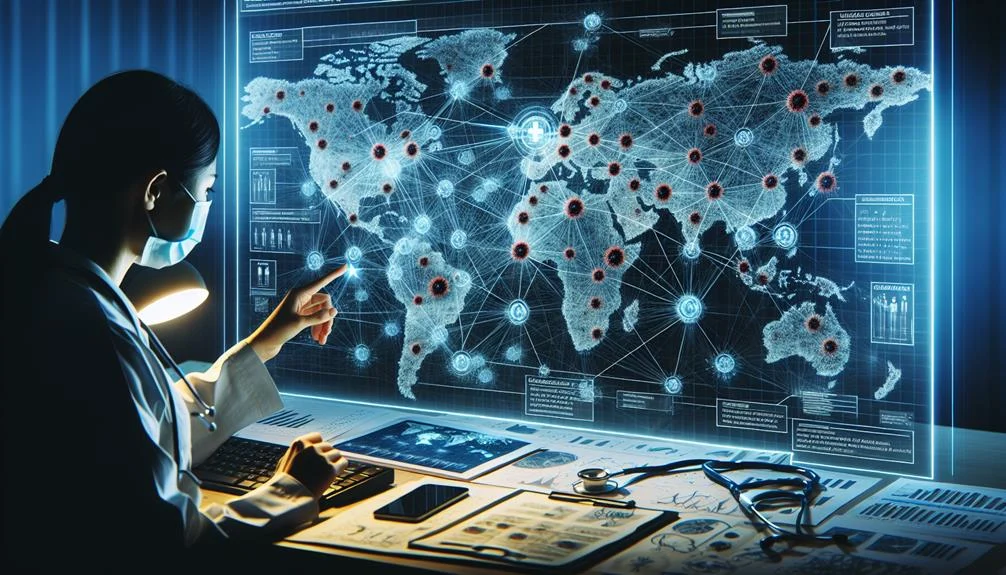
Disease tracking and prevention in healthcare involves the analysis of patterns and trends to predict outbreaks and implement targeted interventions for best public health management. Utilizing data science, healthcare professionals monitor health data trends, track epidemiological data, and develop strategies for disease prevention.
By identifying disease patterns and utilizing data-driven approaches, public health systems can effectively predict outbreaks and respond promptly with preventive measures to minimize their impact on public health.
Data science plays an essential role in disease surveillance by providing real-time insights into the spread of infections, guiding public health policies, and implementing tailored interventions for at-risk populations. Through the use of data-driven strategies, healthcare systems can enhance their capacity to track, prevent, and control diseases, ultimately contributing to improved public health outcomes.
Data Science in Healthcare: Patient Health Record Management
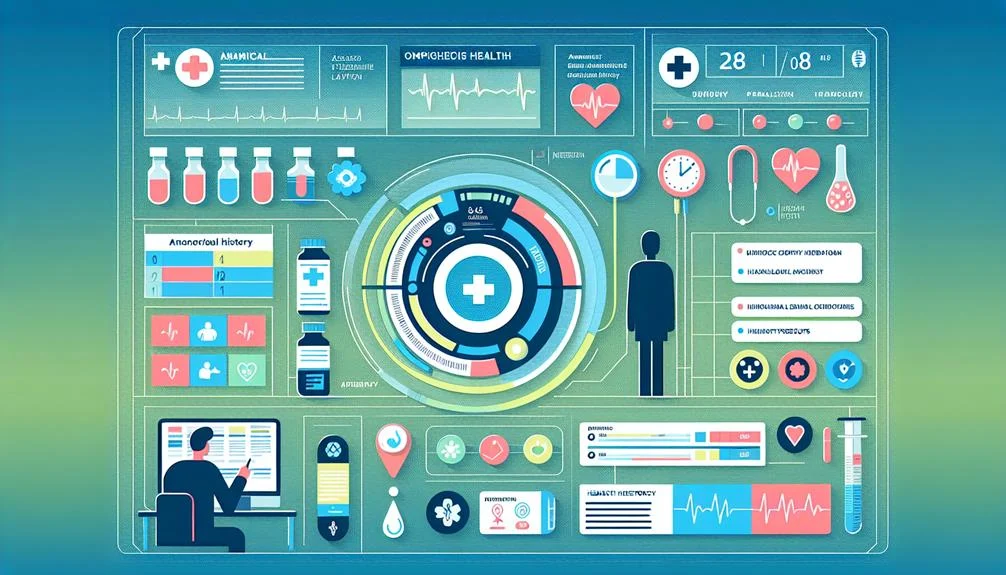
Patient health record management is a critical component of healthcare delivery, enabling the efficient organization and retrieval of medical information for individual patients. Healthcare Data Science plays a pivotal role in this process by utilizing Electronic Health Records (EHRs) to collect, store, and update patient medical records.
Through data-driven decision-making, healthcare providers can access patient information swiftly, fostering seamless communication and collaboration among different care teams. This streamlined approach not only improves patient care but also enhances the overall quality of healthcare services.
Conclusion
In the intricate web of healthcare, data science serves as the guiding light, illuminating the path to improved patient outcomes and enhanced decision-making.
Like a skilled surgeon wielding a scalpel, data-driven algorithms cut through the complexity of medical data, revealing insights that shape the future of healthcare.
With predictive modeling as its compass and disease tracking as its map, data science navigates the vast healthcare landscape with precision and purpose, paving the way for a healthier tomorrow.